What is an AI?
ABOUT AI
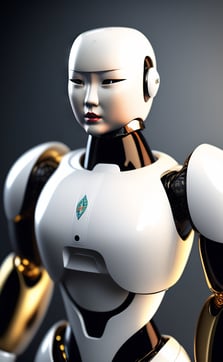
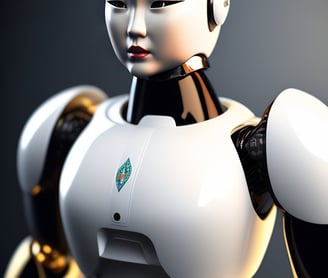
Artificial Intelligence (AI) refers to the development of computer systems or machines that can perform tasks that typically require human intelligence. AI enables machines to simulate cognitive functions such as learning, problem-solving, understanding natural language, recognizing patterns, and making decisions. The goal of AI is to create systems that can perform tasks independently, adapt to new situations, and improve over time without human intervention.
Artificial Intelligence (AI) refers to the development of computer systems or machines that can perform tasks that typically require human intelligence. AI enables machines to simulate cognitive functions such as learning, problem-solving, understanding natural language, recognizing patterns, and making decisions. The goal of AI is to create systems that can perform tasks independently, adapt to new situations, and improve over time without human intervention.
Components of AI:
Machine Learning: One of the core components of AI, machine learning involves training machines to learn from data. It enables algorithms to identify patterns, make predictions, and improve their performance over time.
Deep Learning: Deep learning is a subset of machine learning that uses neural networks to model high-level abstractions in data. These networks learn to perform tasks by considering examples, typically without being explicitly programmed.
Natural Language Processing (NLP): NLP enables machines to understand, interpret, and respond to human language. This technology is used in chatbots, language translation, sentiment analysis, and more.
Computer Vision: AI-driven computer vision systems enable machines to interpret and understand visual information from images or videos. This technology is used in facial recognition, object detection, and medical image analysis.
Types of AI:
Narrow or Weak AI: These systems are designed for specific tasks and operate within a limited domain. Examples include virtual assistants like Siri or Alexa, which respond to voice commands, and recommendation systems used by streaming services or e-commerce platforms.
General AI (AGI): General AI refers to machines that have human-like intelligence and can perform any intellectual task that a human can. AGI is theoretical and does not currently exist.
Superintelligent AI: This level of AI surpasses human intelligence and capabilities in virtually every field. It's also a theoretical concept and is not yet achieved.
AI Applications:
Healthcare: AI is used in diagnostics, drug discovery, personalized medicine, and patient care. Machine learning algorithms analyze medical data to assist in disease detection and treatment planning.
Finance: AI is utilized for fraud detection, algorithmic trading, risk assessment, and personalized financial services. AI-powered algorithms analyze large datasets to predict market trends.
Autonomous Vehicles: AI plays a critical role in self-driving cars, enabling vehicles to perceive their environment and make decisions based on that information.
Robotics: AI powers robots in manufacturing, logistics, and healthcare, allowing them to perform tasks efficiently and adapt to changing environments.
Challenges and Ethics:
AI raises ethical concerns related to privacy, job displacement, biases in algorithms, and the potential for AI systems to make autonomous decisions. Ensuring that AI systems are fair, transparent, and aligned with human values is a crucial challenge in AI development.
Advanced AI Techniques:
Reinforcement Learning: This is a subset of machine learning where agents learn to make sequences of decisions. By receiving feedback in the form of rewards or penalties, they optimize their actions to achieve specific goals. This technique is widely used in robotics and game playing AI.
Transfer Learning: It involves applying knowledge learned from one task to another, accelerating the learning process in new domains. It allows models trained on one dataset to apply some learned knowledge to a different but related dataset.
Generative Adversarial Networks (GANs): GANs consist of two neural networks—generative and discriminative—which compete against each other to improve the accuracy of the generated outputs. They're often used in creating synthetic data or images and in fields like art generation.
AI in Various Fields:
Education: AI is transforming education through personalized learning experiences, intelligent tutoring systems, and adaptive learning platforms that cater to individual student needs.
Environmental Conservation: AI and machine learning are used for monitoring and managing environmental resources. For example, in ecology, AI assists in species identification, tracking animal migration, and monitoring deforestation.
Entertainment and Gaming: AI plays a significant role in creating immersive gaming experiences. AI-driven characters in video games and content recommendation systems in entertainment platforms are examples.
Ethical and Social Implications:
AI Bias and Fairness: There's concern about bias in AI systems that could lead to discrimination, especially in decisions related to hiring, lending, and law enforcement.
Privacy Concerns: As AI systems gather and analyze massive amounts of personal data, there are concerns about data privacy and security breaches.
Employment Impact: The rise of automation and AI may lead to job displacement, which requires strategies for re-skilling and up-skilling the workforce.
Regulatory Challenges: Policymakers are working on developing regulations that balance innovation and ethical considerations, ensuring responsible AI development and deployment.
Future of AI:
Explainable AI (XAI): The push for more transparent and interpretable AI models to understand and explain the decisions made by these systems.
AI and Human Collaboration: The focus is shifting towards AI systems that work collaboratively with humans, augmenting their abilities rather than replacing them.
Continued Advancements: Ongoing research in AI is exploring avenues such as quantum computing, neuro-symbolic AI, and federated learning, among others, to push the boundaries of what AI can achieve.
Advanced AI Techniques:
Neuro-symbolic Computing: This emerging field integrates symbolic reasoning and neural network approaches, aiming to combine the strengths of both to enhance AI's reasoning capabilities.
Explainable AI (XAI): XAI focuses on developing AI models that provide explanations for their decisions, making them more transparent and interpretable, crucial for critical applications like healthcare or finance.
AI Ethics and Governance: As AI becomes more pervasive, the need for ethical guidelines and governance models to ensure responsible and fair AI adoption becomes increasingly critical.
AI in Diverse Fields:
Energy Sector: AI is used for optimizing energy consumption, predictive maintenance in power grids, and facilitating renewable energy integration for better efficiency.
Agriculture: AI applications in precision agriculture include crop monitoring, yield prediction, and optimizing resource usage, contributing to sustainable farming practices.
Space Exploration: AI aids in analyzing vast amounts of data from satellites and telescopes, helping in discovering exoplanets, planning missions, and understanding the universe.
AI and Healthcare:
Drug Discovery: AI accelerates the process of drug discovery by predicting molecular structures and their potential impact, leading to faster innovation in pharmaceuticals.
Personalized Medicine: AI analyzes individual patient data to tailor treatments and therapies according to specific genetic, environmental, and lifestyle factors.
Medical Imaging: AI-powered image analysis assists in the interpretation of medical images, aiding in early diagnosis and treatment planning.
AI Ethics and Societal Impact:
Responsible AI Development: There's a growing emphasis on developing AI that aligns with ethical guidelines, ensuring fairness, accountability, and transparency.
AI and Job Evolution: While AI can automate repetitive tasks, it also creates new job opportunities, particularly in AI development, data science, and AI-assisted roles.
AI Governance and Regulations: Policymakers are working on establishing regulatory frameworks to balance innovation and ethical considerations in AI development and deployment.
Future Implications:
Human-AI Collaboration: The future envisions AI as a tool to augment human abilities, fostering collaboration between humans and machines for enhanced productivity and problem-solving.
AI for Social Good: Increased focus on using AI for addressing societal challenges such as climate change, healthcare accessibility, and education equity.
AI in Creativity: Advancements in AI-driven creative tools in music, art, and literature are being explored, where machines collaborate with human creators.
Advanced AI Techniques:
Federated Learning: This approach trains an AI model across multiple decentralized edge devices or servers while keeping the data on the local device. It's particularly useful in maintaining privacy for sensitive data.
Meta-Learning: Meta-learning explores algorithms that enable AI models to learn how to learn. It involves learning different tasks and leveraging that knowledge to adapt to new, unseen tasks.
AI Explainability: There's a growing focus on understanding and explaining AI models' decisions, especially in critical domains like healthcare and law, where transparency is crucial.
AI in Industry Applications:
Cybersecurity: AI is used for threat detection, identifying patterns in data to predict and prevent cyberattacks, as well as aiding in the rapid response to security breaches.
Customer Service: Chatbots and virtual assistants are extensively used to handle customer queries and improve user experience by providing quick and accurate responses.
Supply Chain Management: AI helps in optimizing supply chain operations by predicting demand, managing inventory, and streamlining logistics.
AI and Climate Change:
Climate Modeling: AI assists in analyzing complex climate data, contributing to more accurate climate models and predictions.
Energy Optimization: AI is utilized in energy-intensive industries to optimize energy usage and increase energy efficiency, thus reducing the environmental impact.
Natural Disaster Prediction: AI algorithms aid in predicting natural disasters like earthquakes, floods, and hurricanes, enabling early warnings and disaster management.
AI Governance and Ethical Considerations:
AI Regulation: Policymakers are working on establishing frameworks to regulate AI development and deployment to ensure safety, fairness, and transparency.
Ethical AI Development: Emphasis is placed on developing AI that respects human values and ethics, ensuring fairness and preventing bias.
Data Privacy: With the proliferation of AI, ensuring data privacy and security becomes paramount to protect individuals' sensitive information.
Future of AI:
AI Democratization: Efforts are being made to make AI more accessible and understandable to non-experts, allowing more people to leverage its power.
AI-driven Creativity: The exploration of AI in creative fields such as music composition, art generation, and writing is expanding, where machines collaborate with human creators.
Continued Advancements: Ongoing research aims at enhancing AI capabilities, such as making systems more adaptable, context-aware, and adept at real-world problem-solving.
Advanced AI Techniques:
Quantum Computing in AI: Exploring the potential for quantum computers to significantly enhance AI capabilities through faster processing, improved algorithms, and solving more complex problems.
Neuroevolution: It's an area that employs evolutionary algorithms to develop neural networks, aiming to improve network architecture and optimize deep learning models.
AI and Robotics Integration: Advancements in AI-driven robotics that focus on enhancing collaboration between robots and humans, particularly in industries like manufacturing, healthcare, and exploration.
AI in Emerging Sectors:
Edge AI: This involves deploying AI algorithms directly on edge devices (such as sensors, IoT devices, or smartphones) rather than relying on a centralized cloud, enabling faster processing and real-time decision-making.
AI in Sports Analytics: Utilizing AI for analyzing player performance, predicting game outcomes, and enhancing strategies in sports like soccer, basketball, and tennis.
AI in Fashion and Design: AI-driven tools that aid in designing, trend prediction, and personalized recommendations in the fashion industry.
AI and Social Impact:
AI for Accessibility: Development of AI tools and technologies to enhance accessibility for people with disabilities, including assistive technologies and adaptive systems.
AI in Mental Health: Applications of AI in analyzing mental health data to improve diagnostics, personalized therapy, and mental health support.
AI in Education Equity: Leveraging AI to create adaptive learning systems, personalized education, and addressing educational disparities.
AI Governance and Ethics:
AI Bias Mitigation: Measures and techniques to reduce bias in AI systems, ensuring fairness and preventing discrimination.
AI Regulation and Standards: Developing regulatory frameworks and industry standards to ensure ethical AI development, deployment, and accountability.
Transparent and Interpretable AI: Focus on creating AI models that can provide explanations and justifications for their decisions, increasing their transparency.
Future Perspectives:
AI Collaboration and Augmentation: Advancements focus on AI as a collaborative tool that augments human capabilities rather than replacing them.
AI Creativity and Innovation: Exploring AI's potential in fostering creativity and innovation across various domains, including art, music, and scientific discoveries.
AI for Global Challenges: Leveraging AI to address significant global challenges such as climate change, healthcare accessibility, poverty, and sustainability.
Advanced AI Techniques:
Adversarial Machine Learning: Research focusing on creating AI models that can withstand attacks and are more robust against adversarial attempts to manipulate or deceive them.
Explainable AI (XAI): Development of AI systems that provide understandable explanations for their decisions, crucial in critical domains where transparency is necessary.
Swarm Intelligence: Emulating the collective behavior of natural systems, like swarms of insects or schools of fish, to develop AI that solves complex problems by working collaboratively.
AI in Evolving Sectors:
AI in Journalism: Utilizing AI for data-driven journalism, content generation, and news curation, aiding in fact-checking and enhancing news coverage.
AI in Agriculture Robotics: Developing AI-powered robots for precision farming, crop monitoring, and autonomous operations in agriculture for increased efficiency.
AI in Mental Well-being Apps: AI-driven mental health apps offering personalized support, tracking emotional well-being, and providing coping strategies.
AI in Ethical and Societal Contexts:
AI and Human Rights: Addressing ethical concerns by ensuring AI respects human rights, does not perpetuate biases, and supports fairness and inclusivity.
AI and Environmental Impact: Leveraging AI to analyze and tackle environmental challenges, such as climate change, biodiversity conservation, and sustainable development.
AI and Racial Bias Mitigation: Developing strategies to identify and remove biases in AI algorithms, ensuring fairness and equity in decision-making processes.
Future Trends and Implications:
Responsible AI Education: Training programs focused on ethical AI development, emphasizing the importance of responsible innovation.
AI and Legal Frameworks: Developing legal frameworks to regulate AI applications, ensuring compliance with ethical and legal standards.
AI in Space Exploration: Using AI for autonomous space missions, navigation, and data analysis in space exploration and research.